Q1. What do you mean by Deep Learning?
Deep Learning is nothing but a paradigm of machine learning which has shown incredible promise in recent years. This is because of the fact that Deep Learning shows a great analogy with the functioning of the human brain.
Q2. What is the difference between machine learning and deep learning?
Machine learning is a field of computer science that gives computers the ability to learn without being explicitly programmed. Machine learning can be categorised in the following three categories.
- Supervised machine learning,
- Unsupervised machine learning,
- Reinforcement learning
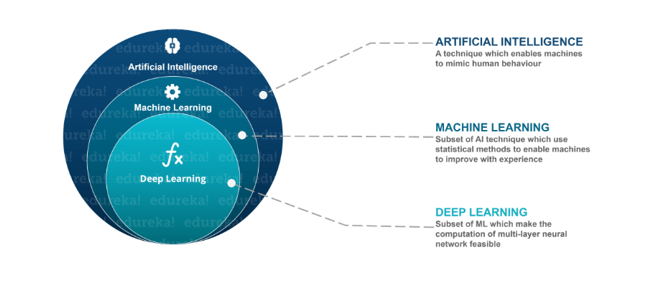
Q3. What, in your opinion, is the reason for the popularity of Deep Learning in recent times?
Now although Deep Learning has been around for many years, the major breakthroughs from these techniques came just in recent years. This is because of two main reasons:
- The increase in the amount of data generated through various sources
- The growth in hardware resources required to run these models
GPUs are multiple times faster and they help us build bigger and deeper deep learning models in comparatively less time than we required previously.
Q4. What is reinforcement learning?
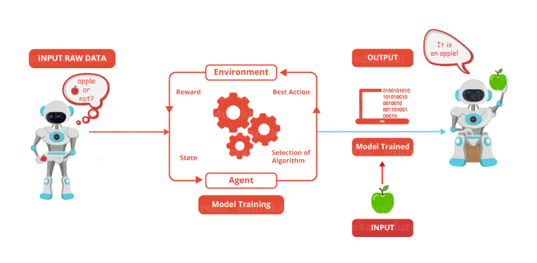
Q5. What are Artificial Neural Networks?
Artificial Neural networks are a specific set of algorithms that have revolutionized machine learning. They are inspired by biological neural networks. Neural Networks can adapt to changing the input so the network generates the best possible result without needing to redesign the output criteria.
Q6. Describe the structure of Artificial Neural Networks?
Artificial Neural Networks works on the same principle as a biological Neural Network. It consists of inputs which get processed with weighted sums and Bias, with the help of Activation Functions.
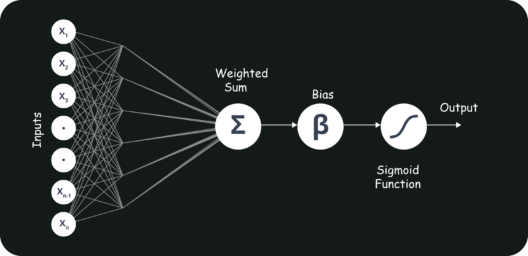
Q7. How Are Weights Initialized in a Network?
There are two methods here: we can either initialize the weights to zero or assign them randomly.Initializing all weights to 0: This makes your model similar to a linear model. All the neurons and every layer perform the same operation, giving the same output and making the deep net useless.
Initializing all weights randomly: Here, the weights are assigned randomly by initializing them very close to 0. It gives better accuracy to the model since every neuron performs different computations. This is the most commonly used method.
Q8. What Is the Cost Function?
Also referred to as “loss” or “error,” cost function is a measure to evaluate how good your model’s performance is. It’s used to compute the error of the output layer during backpropagation. We push that error backwards through the neural network and use that during the different training functions.
Q9. What Are Hyperparameters?
With neural networks, you’re usually working with hyperparameters once the data is formatted correctly. A hyperparameter is a parameter whose value is set before the learning process begins. It determines how a network is trained and the structure of the network (such as the number of hidden units, the learning rate, epochs, etc.).
Q10. What Will Happen If the Learning Rate Is Set inaccurately (Too Low or Too High)?
When your learning rate is too low, training of the model will progress very slowly as we are making minimal updates to the weights. It will take many updates before reaching the minimum point.
If the learning rate is set too high, this causes undesirable divergent behaviour to the loss function due to drastic updates in weights. It may fail to converge (model can give a good output) or even diverge (data is too chaotic for the network to train).
Q11. What Is the Difference Between Epoch, Batch, and Iteration in Deep Learning?
- Epoch – Represents one iteration over the entire dataset (everything put into the training model).
- Batch – Refers to when we cannot pass the entire dataset into the neural network at once, so we divide the dataset into several batches.
- Iteration – if we have 10,000 images as data and a batch size of 200. then an epoch should run 50 iterations (10,000 divided by 50).
Q12. What Are the Different Layers on CNN?
There are four layers in CNN:
- Convolutional Layer – the layer that performs a convolutional operation, creating several smaller picture windows to go over the data.
- ReLU Layer – it brings non-linearity to the network and converts all the negative pixels to zero. The output is a rectified feature map.
- Pooling Layer – pooling is a down-sampling operation that reduces the dimensionality of the feature map.
- Fully Connected Layer – this layer recognizes and classifies the objects in the image.
Q13. What Is Pooling on CNN, and How Does It Work?
Pooling is used to reduce the spatial dimensions of a CNN. It performs down-sampling operations to reduce the dimensionality and creates a pooled feature map by sliding a filter matrix over the input matrix.
Q14. What are Recurrent Neural Networks(RNNs)?
RNNs are a type of artificial neural networks designed to recognise the pattern from the sequence of data such as Time series, stock market and government agencies etc. To understand recurrent nets, first, you have to understand the basics of feedforward nets.
Both these networks RNN and feed-forward named after the way they channel information through a series of mathematical orations performed at the nodes of the network. One feeds information through straight(never touching the same node twice), while the other cycles it through a loop, and the latter are called recurrent.
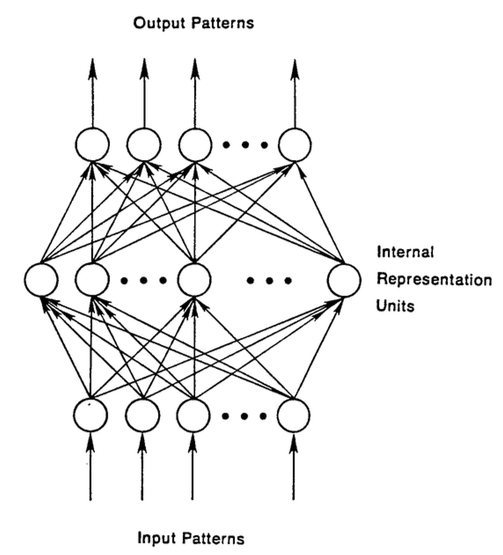
Recurrent networks, on the other hand, take as their input, not just the current input example they see, but also the what they have perceived previously in time.
The decision a recurrent neural network reached at time t-1 affects the decision that it will reach one moment later at time t. So recurrent networks have two sources of input, the present and the recent past, which combine to determine how they respond to new data, much as we do in life.
The error they generate will return via backpropagation and be used to adjust their weights until error can’t go any lower. Remember, the purpose of recurrent nets is to accurately classify sequential input. We rely on the backpropagation of error and gradient descent to do so.
Q15. How Does an LSTM Network Work?
Long-Short-Term Memory (LSTM) is a special kind of recurrent neural network capable of learning long-term dependencies, remembering information for long periods as its default behaviour. There are three steps in an LSTM network:
- Step 1: The network decides what to forget and what to remember.
- Step 2: It selectively updates cell state values.
- Step 3: The network decides what part of the current state makes it to the output.
Q16. What Is a Multi-layer Perceptron(MLP)?
As in Neural Networks, MLPs have an input layer, a hidden layer, and an output layer. It has the same structure as a single layer perceptron with one or more hidden layers. A single layer perceptron can classify only linear separable classes with binary output (0,1), but MLP can classify nonlinear classes.
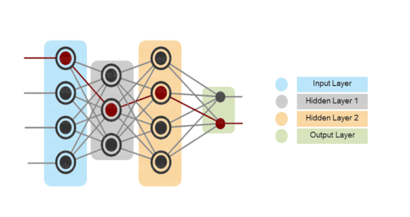
Q17. Explain Gradient Descent.
To Understand Gradient Descent, Let’s understand what is a Gradient first.
A gradient measures how much the output of a function changes if you change the inputs a little bit. It simply measures the change in all weights with regard to the change in error. You can also think of a gradient as the slope of a function.
Gradient Descent can be thought of climbing down to the bottom of a valley, instead of climbing up a hill. This is because it is a minimization algorithm that minimizes a given function (Activation Function).
Q18. What is exploding gradients?
While training an RNN, if you see exponentially growing (very large) error gradients which accumulate and result in very large updates to neural network model weights during training, they’re known as exploding gradients. At an extreme, the values of weights can become so large as to overflow and result in NaN values.
This has the effect of your model is unstable and unable to learn from your training data.
Q19. What is vanishing gradients?
While training an RNN, your slope can become either too small; this makes the training difficult. When the slope is too small, the problem is known as a Vanishing Gradient. It leads to long training times, poor performance, and low accuracy.
Q20. What is Back Propagation and Explain it’s Working.
Backpropagation is a training algorithm used for multilayer neural network. In this method, we move the error from an end of the network to all weights inside the network and thus allowing efficient computation of the gradient.
It has the following steps:
- Forward Propagation of Training Data
- Derivatives are computed using output and target
- Back Propagate for computing derivative of error wrt output activation
- Using previously calculated derivatives for output
- Update the Weights
Q21. What are the variants of Back Propagation?
- Stochastic Gradient Descent: We use only a single training example for calculation of gradient and update parameters.
- Batch Gradient Descent: We calculate the gradient for the whole dataset and perform the update at each iteration.
- Mini-batch Gradient Descent: It’s one of the most popular optimization algorithms. It’s a variant of Stochastic Gradient Descent and here instead of single training example, mini-batch of samples is used.
Q22. What are the different Deep Learning Frameworks?
- Pytorch
- TensorFlow
- Microsoft Cognitive Toolkit
- Keras
- Caffe
- Chainer
Q23. What is the role of the Activation Function?
The Activation function is used to introduce non-linearity into the neural network helping it to learn more complex function. Without which the neural network would be only able to learn linear function which is a linear combination of its input data. An activation function is a function in an artificial neuron that delivers an output based on inputs.
Q24. Name a few Machine Learning libraries for various purposes.
Q25. What is an Auto-Encoder?
Auto-encoders are simple learning networks that aim to transform inputs into outputs with the minimum possible error. This means that we want the output to be as close to input as possible. We add a couple of layers between the input and the output, and the sizes of these layers are smaller than the input layer. The auto-encoder receives unlabelled input which is then encoded to reconstruct the input.
Q26. What is a Boltzmann Machine?
Boltzmann machines have a simple learning algorithm that allows them to discover interesting features that represent complex regularities in the training data. The Boltzmann machine is basically used to optimise the weights and the quantity for the given problem. The learning algorithm is very slow in networks with many layers of feature detectors. “Restricted Boltzmann Machines” algorithm has a single layer of feature detectors which makes it faster than the rest.
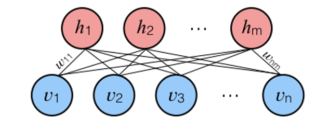
Dropout is a technique of dropping out hidden and visible units of a network randomly to prevent overfitting of data (typically dropping 20 per cent of the nodes). It doubles the number of iterations needed to converge the network.
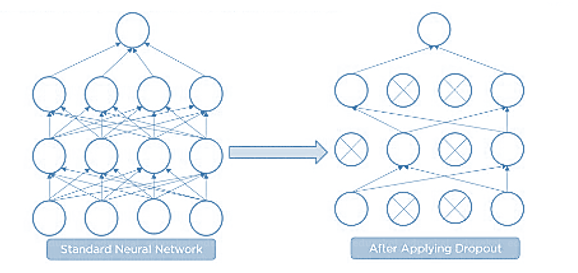
Batch normalization is the technique to improve the performance and stability of neural networks by normalizing the inputs in every layer so that they have mean output activation of zero and standard deviation of one.
Q28. What Is the Difference Between Batch Gradient Descent and Stochastic Gradient Descent?
Batch Gradient Descent
|
Stochastic Gradient Descent
|
The batch gradient computes the gradient using the entire dataset.
|
The stochastic gradient computes the gradient using a single sample.
|
It takes time to converge because the volume of data is huge, and weights update slowly.
|
It converges much faster than the batch gradient because it updates weight more frequently.
|
Q29. Why Is Tensorflow the Most Preferred Library in Deep Learning?
Tensorflow provides both C++ and Python APIs, making it easier to work on and has a faster compilation time compared to other Deep Learning libraries like Keras and Torch. Tensorflow supports both CPU and GPU computing devices.
Q30. What Do You Mean by Tensor in Tensorflow?
A tensor is a mathematical object represented as arrays of higher dimensions. These arrays of data with different dimensions and ranks fed as input to the neural network are called “Tensors.”
Q31. What is the Computational Graph?
Everything in a tensorflow is based on creating a computational graph. It has a network of nodes where each node operates, Nodes represent mathematical operations, and edges represent tensors. Since data flows in the form of a graph, it is also called a “DataFlow Graph.”
Q32. What is a Generative Adversarial Network?
Suppose there is a wine shop purchasing wine from dealers, which they resell later. But some dealers sell fake wine. In this case, the shop owner should be able to distinguish between fake and authentic wine.
The forger will try different techniques to sell fake wine and make sure specific techniques go past the shop owner’s check. The shop owner would probably get some feedback from wine experts that some of the wine is not original. The owner would have to improve how he determines whether a wine is fake or authentic.
The forger’s goal is to create wines that are indistinguishable from the authentic ones while the shop owner intends to tell if the wine is real or not accurately
Let us understand this example with the help of an image.
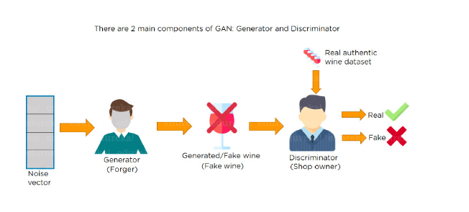
Here the forger acts as a Generator.
The shop owner acts as a Discriminator.
The Discriminator gets two inputs; one is the fake wine, while the other is the real authentic wine. The shop owner has to figure out whether it is real or fake.
So, there are two primary components of Generative Adversarial Network (GAN) named:
- Generator
- Discriminator
The generator is a CNN that keeps keys producing images and is closer in appearance to the real images while the discriminator tries to determine the difference between real and fake images The ultimate aim is to make the discriminator learn to identify real and fake images.
Apart from the very technical questions, your interviewer could even hit you up with a few simple ones to check your overall confidence, in the likes of the following.
Q33. What are the important skills to have in Python with regard to data analysis?
The following are some of the important skills to possess which will come handy when performing data analysis using Python.
- Good understanding of the built-in data types especially lists, dictionaries, tuples, and sets.
- Mastery of N-dimensional NumPy Arrays.
- Mastery of Pandas dataframes.
- Ability to perform element-wise vector and matrix operations on NumPy arrays.
- Knowing that you should use the Anaconda distribution and the conda package manager.
- Familiarity with Scikit-learn. **Scikit-Learn Cheat Sheet**
- Ability to write efficient list comprehensions instead of traditional for loops.
- Ability to write small, clean functions (important for any developer), preferably pure functions that don’t alter objects.
- Knowing how to profile the performance of a Python script and how to optimize bottlenecks.
Reference : Thanks to Edureka for an awesome collection
Comments
Post a Comment