Recently I was trying to create Conda Environment and wanted to install Tensorflow but i have faced some issue , so i have done some research and done trouble shooting related to that . Here am going to share how to trouble shoot if you are getting Conda Value error while creating Conda environment and install tensorflow
.
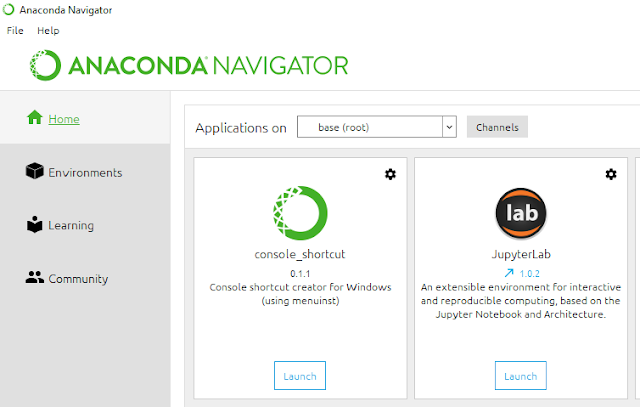
Launch the console from Anaconda Navigator and
conda create -n mypython python=3.6.8
After Installing Conda environment please active the conda now : conda activate mypython
once conda environment has been activated kindly install tensorflow 2.0 by using this command pip install tensorflow==2.0.0
once Tensorflow has been successfully install kindly run the command : pip show tensorflow
.
- Open Anaconda Prompt (as
administrator if it was installed for all users)
- Run conda update conda
- Run the installer again
Make
sure all pkg are updated:
Launch the console from Anaconda Navigator and
conda create -n mypython python=3.6.8
After Installing Conda environment please active the conda now : conda activate mypython
once conda environment has been activated kindly install tensorflow 2.0 by using this command pip install tensorflow==2.0.0
once Tensorflow has been successfully install kindly run the command : pip show tensorflow
Try to Run Comman PIP Install Jupyter lab and after installing launch the console and open Jupyter notebook
Happy Learning...!!
Thanks Anand its really help and guide me solve very similar issue.
ReplyDelete